Wednesday, 23 April 2025
Creating a redress scheme in an AI-powered environment
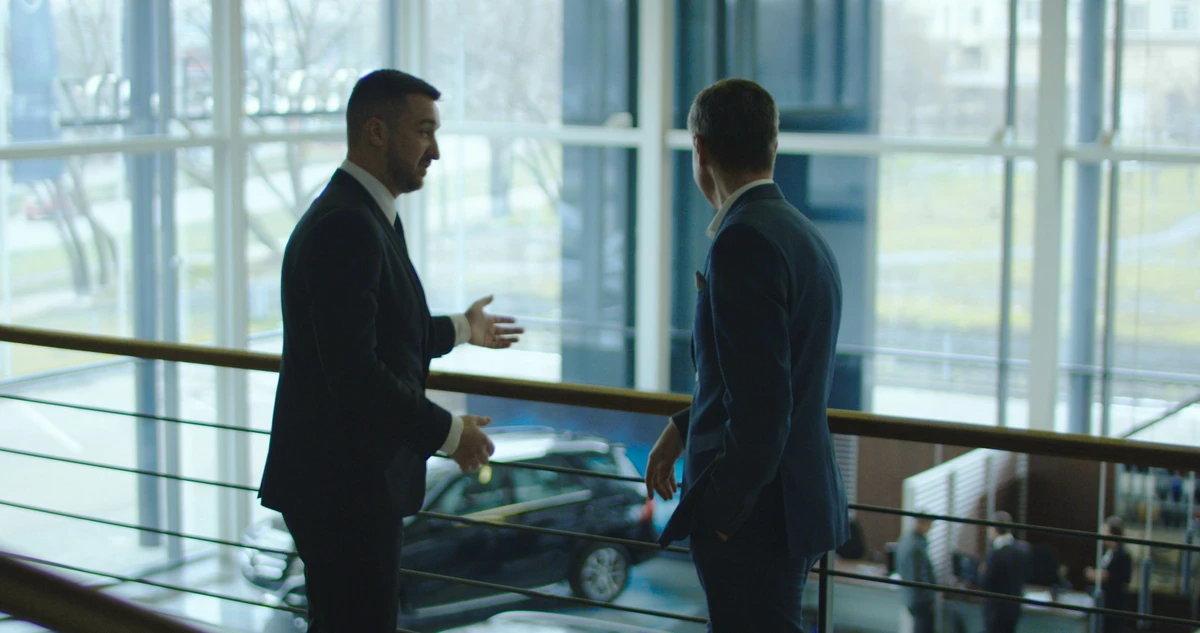
In the wake of the appeal hearing by the Supreme Court in early April, motor finance providers are waiting to learn whether and how they must offer financial compensation to borrowers who were mis-sold car finance.
The appeal, brought by lenders Close Brothers and FirstRand Bank, challenged a 2024 Court of Appeal ruling, which found it unlawful for car dealers to receive commissions from lenders without securing the customer’s fully informed consent.
While the Supreme Court case directly relates to commission disclosure complaints, finance industry regulator, the Financial Conduct Authority (FCA) is also considering the past misuse of car finance discretionary commission arrangements, which incorporated hidden commission. The FCA has said it will report back to the finance sector on next steps within six weeks of the Supreme Court’s decision, which is expected in early summer.
Already, the FCA has said it considers a redress scheme “simpler for consumers than bringing a complaint”. With millions of car buyers possibly needing to be compensated, lenders need to start thinking now about the systems they should be putting in place to drive data accuracy in a redress scheme. Artificial intelligence (AI) should be an essential part of their response.
Across the finance sector, there are examples of AI’s deployment to drive data insights, streamline customer interactions and accelerate decision-making and risk assessment. Around 75 percent of firms in the UK financial services sector are now using AI and that figure is set to grow, research by the Bank of England and FCA revealed late last year. But almost half of respondents to this study said that they had only a “partial understanding” of the AI technologies they were using, largely due to the application of third-party models.
AI technologies and large language models (LLMs) can significantly enhance regulatory compliance in customer remediation projects, but benefits can only be fully realised when technology is deployed effectively. Talan Data brings the specialist know-how to support and empower lenders to deliver a successful remediation project that harnesses the potential of AI.
We outline how AI can be deployed in this third blog in our series giving practical guidance for motor finance providers as they plan for potential remediation projects.
When deploying AI, it is important to pay attention to:
Human oversight
Effective implementations maintain human experts for strategic decisions and edge cases, using AI as a decision support tool
Explainability mechanisms
Systems should provide clear explanations for recommendations to satisfy regulatory transparency requirements
Model governance
Organisations need robust frameworks for testing and validating AI models used in compliance-critical functions.
A key decision for lenders is the level of support needed in developing a remediation project and the extent to which that should be delivered by specialist partners. Potential risks, such as non-compliance, regulatory fines, data security breaches and inaccurate decisions and payouts, can be successfully mitigated by working with expert specialists.
Talan Data’s work is informed by two decades of experience in managing and supporting large-scale remediation programmes, including complex redress and data quality initiatives for the financial services sector. We have a team of data specialists ready to prepare and support responses to an anticipated redress scheme – taking lenders from strategy through to implementation.
Our experience and expertise position us to drive the effective application of AI technologies in remediation projects while enabling benefits for lenders and their customers.
Read our previous Blogs